
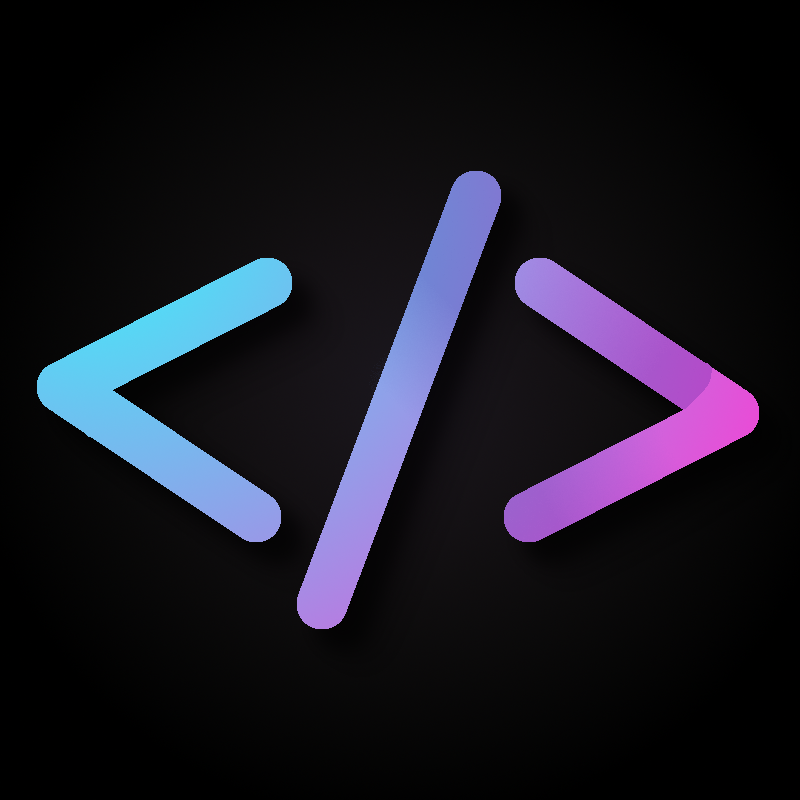
Or I should explain better: most training samples will be cut off at the top, so the network sort of learns to ignore it a bit.
Or I should explain better: most training samples will be cut off at the top, so the network sort of learns to ignore it a bit.
Yes, that’s by design, the networks work on transcripts per input, it does genuinely get cut off eventually, usually it purges an entire older line when the tokens exceed a limit.
I was a curious child, and things spiralled out of control from there…
Found it in a cross post: https://www.nature.com/articles/s41586-023-06668-3
Its a transformer, someone fire the journalist. Still, interesting stuff
Anybody have a link to the paper? The article strikes me as a used car salemans trying to sell me a journal. Mostly what I’m getting is new reinforcement learning technique catered to language? But what model architecture? Is it new? I’d like to know
Maybe a bit late, but I’ve worked on this kind of functionality. I did not work on the algorithm, but the guys who did say:
This is of course based on trust, but these are the claims.
Ah, that makes sense. Most cloud providers have the full nine yards with online hardware provisioning and imaging I forgot you could still just rent a real machine.
Hmm, wonder if there was some reason they didnt just extract the original certificates from the VPS if it was actually the hosting provider, I mean even with mitigation it should be sitting in a temp folder somewhere, surely they could? Issuing new ones seems like a surefire way to alert the operators, unless they already used Let’s Encrypt of course.
They previously did not use APEX but that seems to have changed recently: https://github.com/GrapheneOS/grapheneos.org/commit/7bf9b2671667828d1553c92bf4f64cc749b74d0b Regardless it will need the verified boot keys it seems so Google can’t update them, likely the devs will take responsibility to update the CAs. No idea if they will restore the user control though.
I feel like this is just describing the future of business processing consultants. Like there’s already a role for this, unless I’m missing something?
Oooh looks like it can use a sort of inline Jupyter notebook that’s actually really cool. Hopefully it doesn’t have network access in the sandbox or it can definitely try it’s hand at hacking if asked lol
I think the part that annoys me the most is the hype around it, just like blockchain. People who don’t know any better claiming magic.
We’ve had a few sequence specific architectures over the years. GRU, LSTM and now Transformers. They were all better than the last at the task of sequence specific transformations, and at least for the last one the specific task was language translation. We eventually figured out these guys have a bit of clairvoyance too, they could make accurate predictions based on past data, or at least accurate enough to bet on, and you can bet traders of various stripes have already made billions off that fact. I’ve even seen a transformer based weather model. It did OK, but transformers are better at language.
And that’s all it is! ChatGPT is a Transformer in the predictive stance. It looks at a transcript of a conversation and thinks what a human is most likely to say next. It’s a very complex transformation of historical data. If you give it the exact same transcript, it gives the exact same answer. It is in the literally mathematically rigorous sense entirely incapable of an original thought. Any perceived sentience is a shadow of OpenAI’s army of annotators or the corpus it was trained on, and I have a hard time assigning sentience to tomorrow’s forecast, which may well have used similar technology. It’s just an ultra fancy search engine index.
Anyways, that’s my rant done I guess. Call it a cynical engineer’s opinion. To be clear I think it’s a fantastic and useful technology, and it WILL change how we interact with machines. It can do fancy things with the combination of “shell” code driving it’s UI like multi-step “agents” or running code, and I actually hope OpenAI extends it far into the future, but I sincerely think any form of AGI will be something entirely different to LLMs, or at least they’ll only form a small part of it as an encoder/decoder for it’s thoughts.
EDIT: Added some paragraph spacing. Sorry, went into a more broad AI rant rather than staying on topic about coding specifically lol
Yeah, in my mind I thought of it more as a “why not” in addition to vision. Like why make it only as capable as the humans its trying to replace when it can have even more data to work with? Probably would have been even more expensive though
True that, he did good on that front for a while though. He got too confident
I remember hearing a while back that Musk made an executive decision at Tesla to not use LIDAR. I thought: “That’s a stupid decision. At least invest in making it better if you think its not sufficient” and I had a quite negative view of his engineering abilities ever since. Seeing as a Tesla can be fooled by a projector these days, I’m willing to die on that hill. I will admit that he is an exceptional businessman, most people would piss away a fortune if given one, but an engineer he is not, not by a loooooooong way.
I’ve not played with it much but does it always describe the image first like that? I’ve been trying to think about how the image input actually works, my personal suspicion is that it uses an off the shelf visual understanding network(think reverse stable diffusion) to generate a description, then just uses GPT normally to complete the response. This could explain the disconnect here where it cant erase what the visual model wrote, but that could all fall apart if it doesn’t always follow this pattern. Just thinking out loud here
Thanks for the detailed reply, I see that I did indeed misunderstand what he was saying. I’m an R&D engineer so I guess my knee jerk response to character level mischief is exactly what you said, it can’t see them anyway, I already knew that so I dismissed that possible interpretation in my mind straight out the gate. Maybe I should assume zero knowledge of internal AI workings reading commentary in the wild.
Edit: Actually just thought of a good analogy for this. Say I play a sound and then ask you what it is of. You might reply “it sounds like a bell”, but if I asked exactly the composition of frequencies that made the sound, you might not be able to say. Similarly the AI sees a group of letters as a definite “thing” (token) but it doesn’t know what actually went into that because its “ears”(tokenizer) already reduced it to a simpler signal.
?? Literally the entire purpose of the transformer architecture is to manipulate text, how is it bad at that? Am I misunderstanding this? Summarization, thematic transformation, language translation etc are all things AI is fantastic at…
Ah, even then it could just be a consequence of training samples usually being chronological(most often the expected resolution for conflicting instructions is “whatever you heard last”, with some exceptions when explicitly stated) so it learns to think that way. I did find the pattern also applies to GPT trained on long articles where you’d expect it not to, so wanted to just explain why that might be.